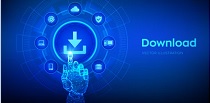
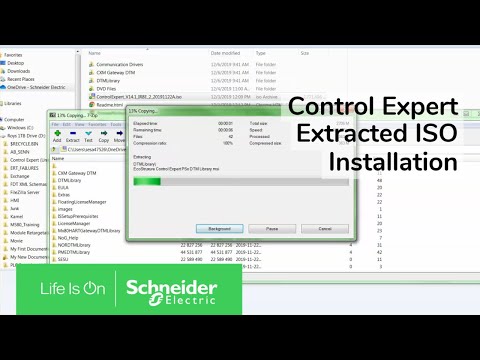

The MCF10A organoid data set (underlying Figs 5, 6, Figs D-F in S1 Text) is 15 GB in size and consists of 99786 single plane images (IDR “screenC"). The excitotoxicity data set (underlying Fig C in S1 Text) is 83 GB in size and consists of 33075 single plane images (IDR “screenB"). The entire multicolor 3D confocal data set for the BH3 mimetics experiments (underlying Figs 3, 4, Fig A in S1 Text) is 2.74 TB in size and consists of 437400 single plane images (IDR “screen A”). This is an open access article distributed under the terms of the Creative Commons Attribution License, which permits unrestricted use, distribution, and reproduction in any medium, provided the original author and source are credited.ĭata Availability: All 3D microscopy data sets generated in this study were deposited to the Open Microscopy Image Data Resource repository ( ) under accession number idr0105. Received: JAccepted: DecemPublished: February 22, 2021Ĭopyright: © 2021 Mergenthaler et al. Saucerman, University of Virginia, UNITED STATES PLoS Comput Biol 17(2):Įditor: Jeffrey J. Our method enables novel opportunities for rapid large-scale multivariate phenotypic microscopy image analysis in 3D using a standard desktop computer.Ĭitation: Mergenthaler P, Hariharan S, Pemberton JM, Lourenco C, Penn LZ, Andrews DW (2021) Rapid 3D phenotypic analysis of neurons and organoids using data-driven cell segmentation-free machine learning. Together these techniques provide the means for facile discovery and interpretation of meaningful patterns in a high dimensional feature space without complex image processing and prior knowledge or assumptions about the feature space. Further, we present methods for analyzing this data by classification or clustering. Here, we report that using data-driven voxel-based features and machine learning it is possible to analyze complex 3D image data without compressing them to 2D, identifying individual cells or using computationally intensive deep learning techniques. However, unbiased quantitative phenotypic analysis of microscopy images of cells grown in 3D organoids or in dense culture conditions in large enough numbers to reach statistical clarity remains a fundamental challenge.
Download cellprofiler analyst software 64 bit code#
Phindr3D is provided as Matlab code and as a stand-alone program ( ).įluorescence microscopy is a fundamental technology for cell biology. Our novel implementation of image analysis algorithms called Phindr3D allowed rapid implementation of data-driven voxel-based feature learning into 3D high content analysis (HCA) operations and constitutes a major practical advance as the computed assignments represent the biology while preserving the heterogeneity of the underlying data. We demonstrate the analysis potential on complex 3D images by investigating the phenotypic alterations of: neurons in response to apoptosis-inducing treatments and morphogenesis for oncogene-expressing human mammary gland acinar organoids. Here we describe a comprehensive shallow-learning framework for automated quantitative phenotyping of three-dimensional (3D) image data using unsupervised data-driven voxel-based feature learning, which enables computationally facile classification, clustering and advanced data visualization.
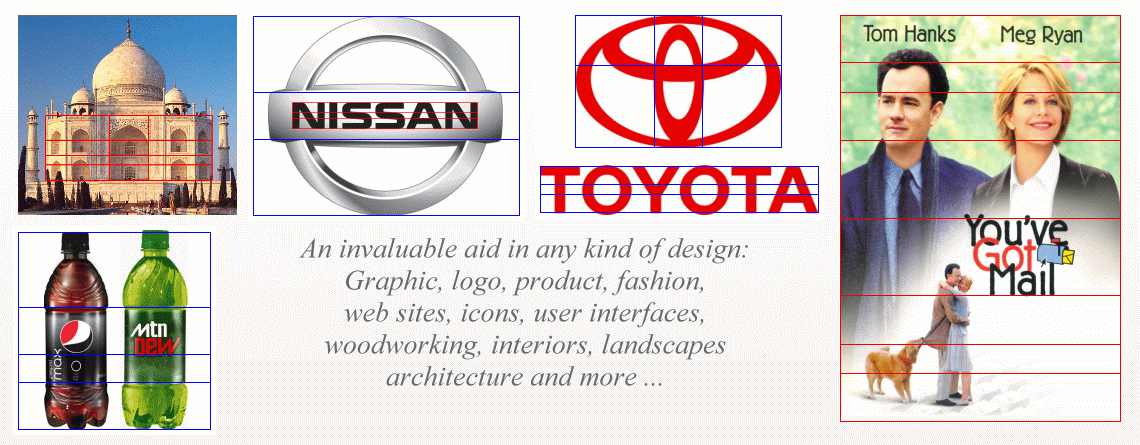
The computational demands of automated processing further limit analysis of hard-to-segment images such as of neurons and organoids. Phenotypic profiling of large three-dimensional microscopy data sets has not been widely adopted due to the challenges posed by cell segmentation and feature selection.
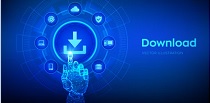